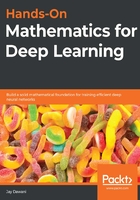
Joint distributions
So far, we have dealt with and learned about distributions that relate to one random variable; but now, say we have two random variables, X and Y. Then, their joint distribution is defined as , such that
.
In joint distributions, we usually tend to know the distribution of a set of variables, but sometimes, we may only want to know the distribution of a subset. We call this the marginal distribution. We define the marginal distribution of X as follows:

Let's say our n continuous random variables in A are jointly distributed and have the f PDF. Then, we have the following:
Here, and
.
Let's revisit an earlier example, where we have two variables, X and Y. If the variables are continuous, then their joint distribution is and
.
Now, let's suppose our n continuous random variables are independent. Then, for all cases of
.
If is a cumulative distribution function and
is the PDF, then
and
.